Polypharmacology |
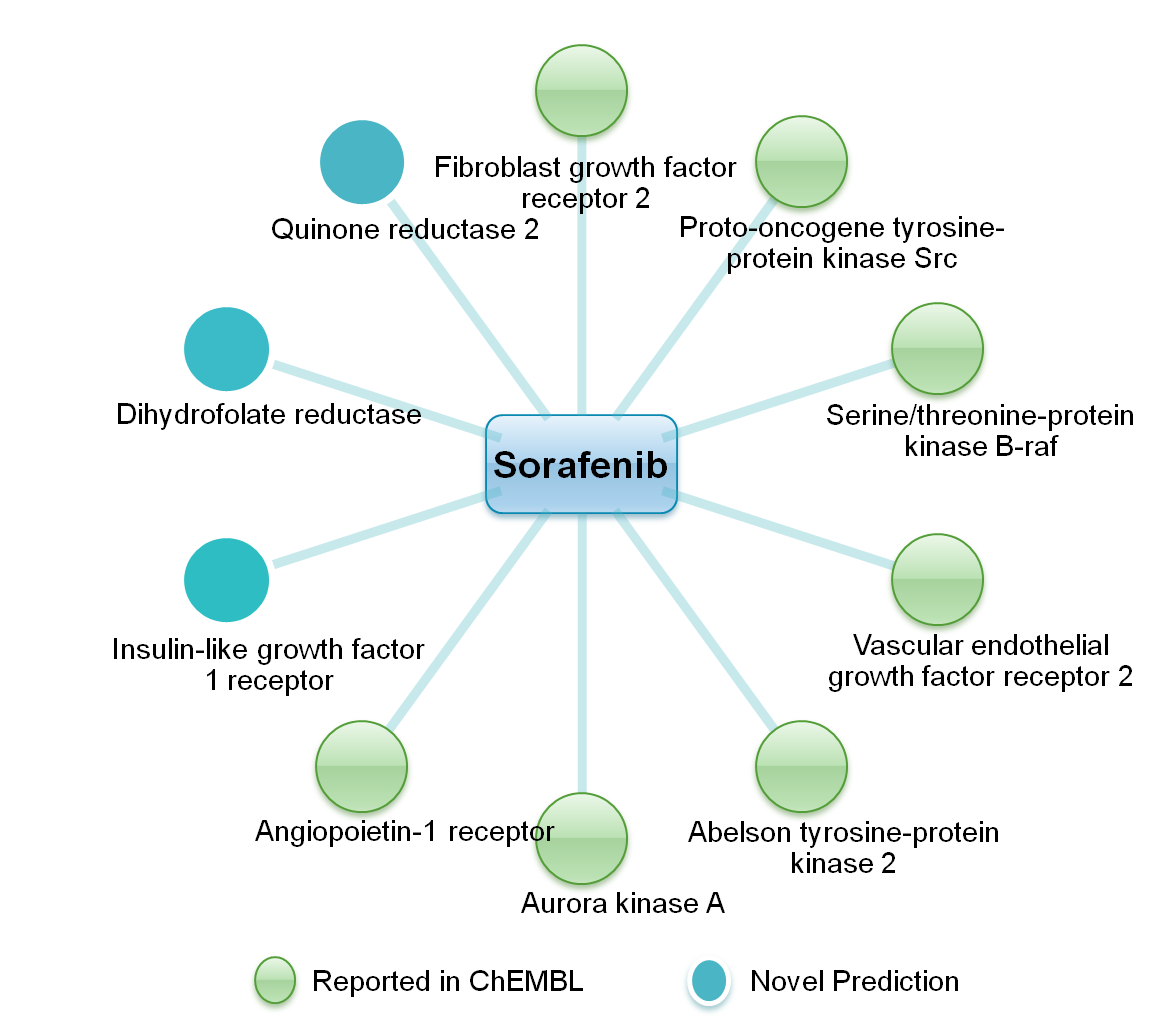 | Proteochemometric (PCM) methods, which use descriptors of both the interacting species, i.e. drug
and the target, are being successfully employed for the prediction of drug–target interactions (DTI).
However, unavailability of non-interacting dataset and determining the applicability domain (AD) of
model are a main concern in PCM modeling. To establish the practical utility of built models, targets
were predicted for approved anticancer drugs of natural origin. The molecular recognition interactions
between the predicted drug–target pair were quantified with the help of a reverse molecular docking
approach. The majority of predicted targets are known for anticancer therapy. These results thus correlate
well with anticancer potential of the selected drugs. Interestingly, out of all predicted DTIs, thirty were
found to be reported in the ChEMBL database, further validating the adopted methodology. |
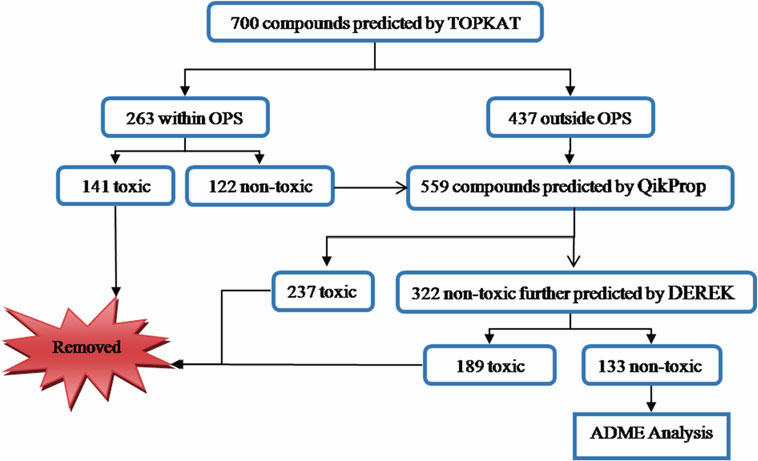 |
Therapeutic agents exert their pharmacological
and adverse effects by interacting with molecular targets.
Even if drug molecules are intended to interact with specific
targets in a desirable manner, they are often found to
bind to other targets. Phloroglucinols represent a
class of compounds, which exhibits a diverse range of biological
activities, such as anti-HIV, antimalarial, antileishmanial,
antituberculosis, antibacterial, and antifungal. The
aim of the current study is to explore untapped potential
of various series of phloroglucinols against HIV reverse
transcriptase (HIV-RTase). A library of phloroglucinol derivatives
was screened based on their toxicity potential followed by
predicted ADME parameters. The filtered compounds were
then carried forward for docking analysis against HIV-RTase.
A set of 37 phloroglucinol compounds with diverse pharmacological
profile was found to have good binding affinity towards HIV-RTase. These molecules formed hydrogen
bonds with Lys101, Lys103, Val106, and Leu234 residues
and p–p stacking interaction with Tyr318 residue of the protein.
|
Kinetoplastids |
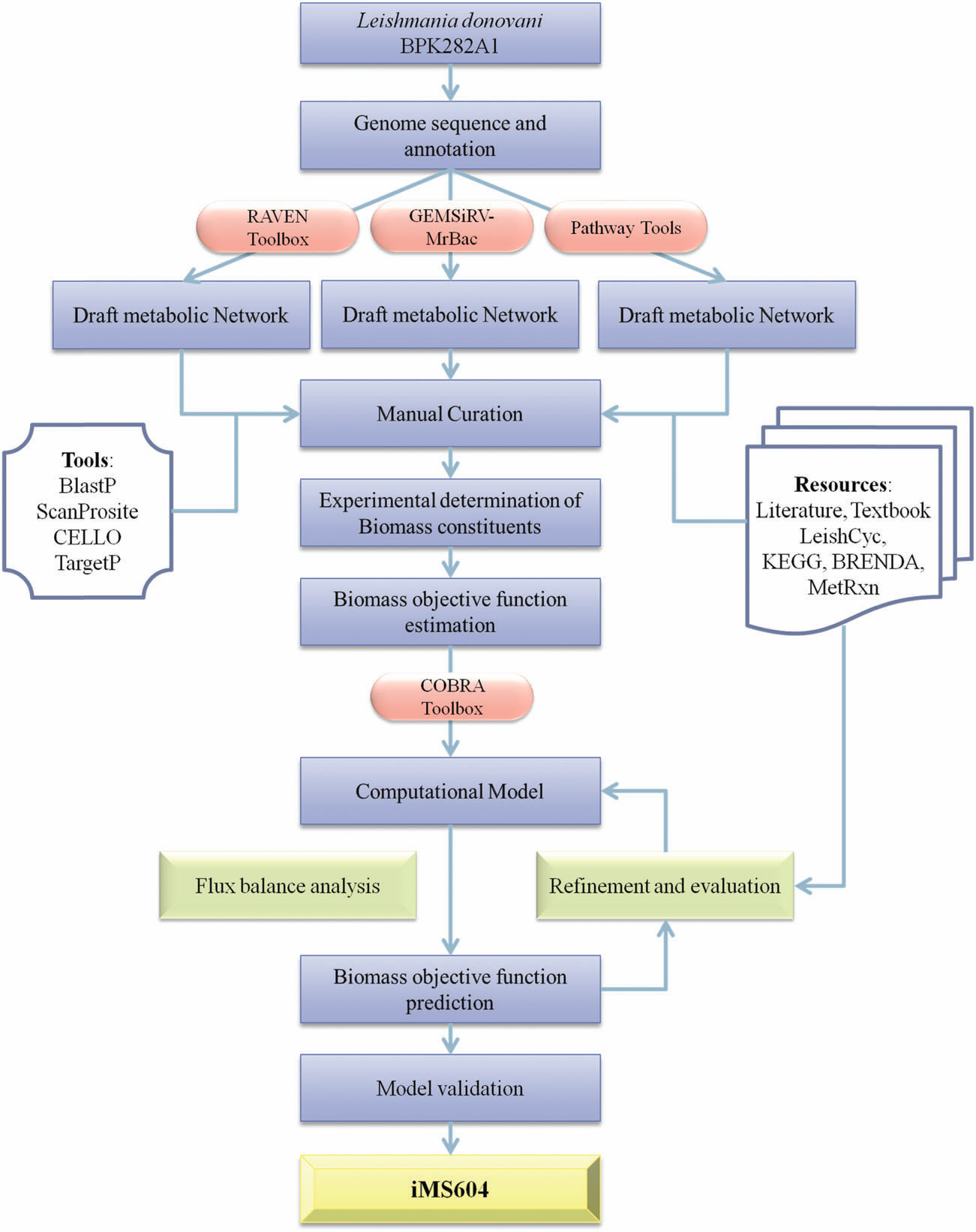 |
Visceral leishmaniasis, a lethal parasitic disease, is caused by the protozoan parasite Leishmania donovani. The
absence of an effective vaccine, drug toxicity and parasite resistance necessitates the identification of novel
drug targets. Reconstruction of genome-scale metabolic models and their simulation has been established
as an important tool for systems-level understanding of a microorganism’s metabolism. In this work, a
constraint-based metabolic model for Leishmania donovani BPK282A1 has been developed. The
developed model is a highly compartmentalized metabolic model, comprising 1159 reactions, 1135
metabolites and 604 genes. The model exhibited around 76% accuracy for the prediction of experimental
phenotypes of gene knockout studies and drug inhibition assays. Employing in silico gene knockout studies,
28 essential genes were identified with negligible sequence identity to the human proteins. |
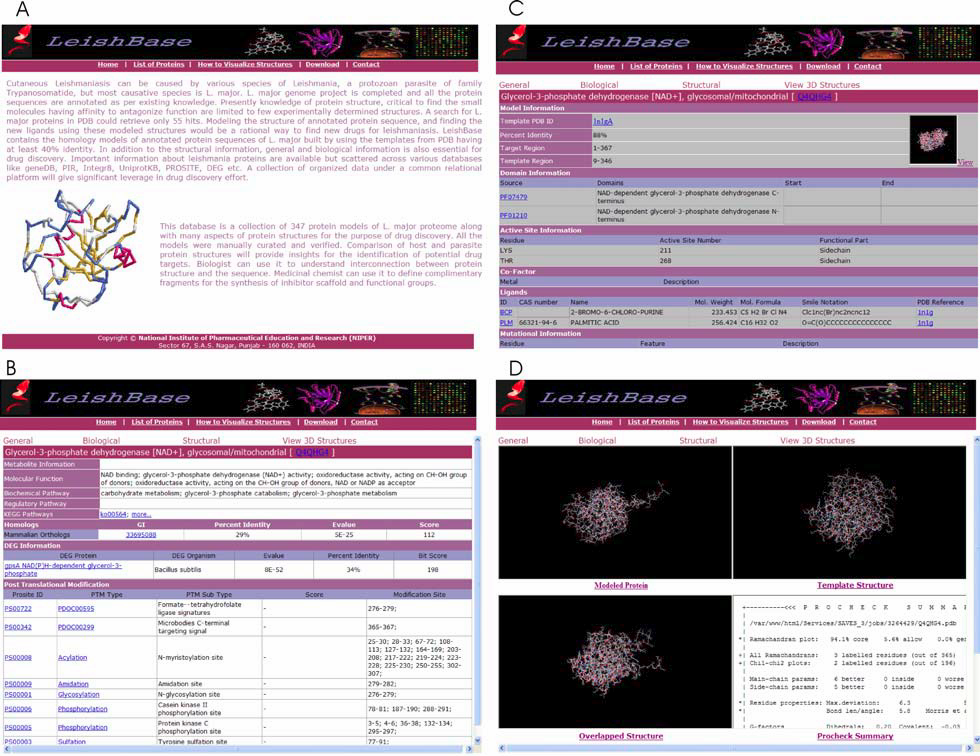 |
LeishBase is a database of 347 Leishmania major proteins whose structures have been modeled by homology modeling. Detailed structural and biological details of Leishmania major proteins can provide a better understanding of their function and a way to identify potential targets involved in disease pathology. Also, an effort has been made to identify and prioritize targets among the 347 proteins present in this database. A list of top 12 targets is provided that may be probable Leishmania major drug targets. A collection of organized data under a common relational platform will give significant leverage in drug discovery effort against Leishmaniasis. |
Tuberculosis |
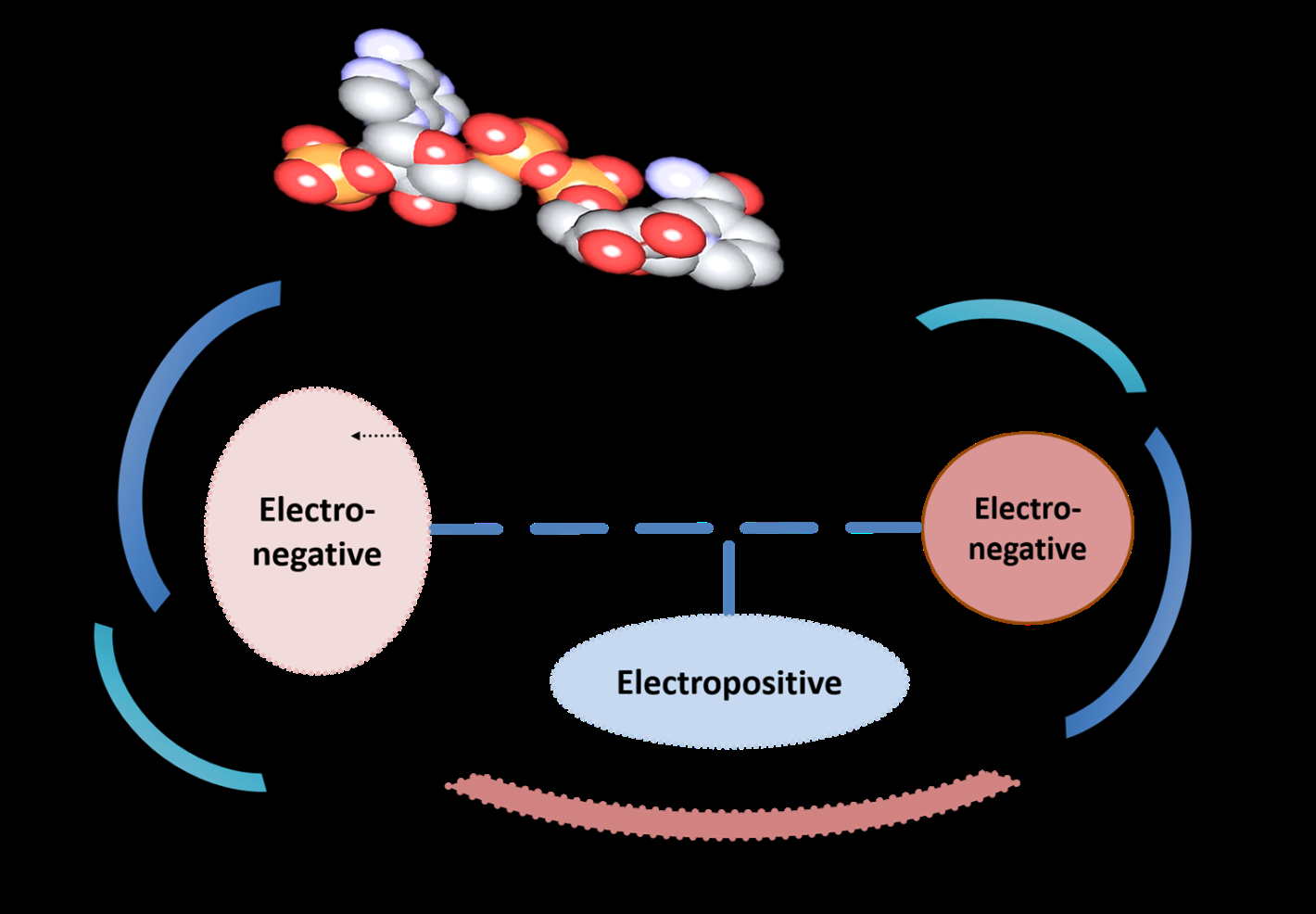 | A combined hypothesis to guide Mtb-ASADH inhibitor design. This hypothesis was arrived at on the basis of the pharmacophoric perception gained in this entire computational work. |
Protein Function |
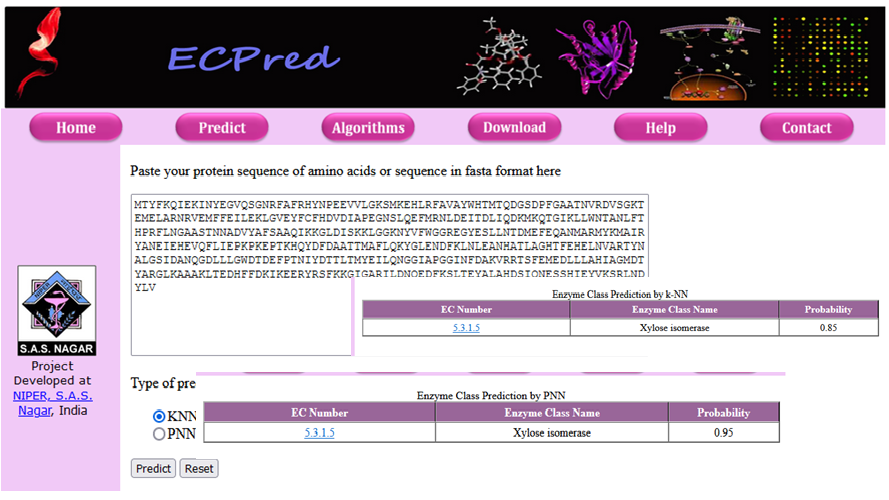 | Functional annotation of protein at genomic scale is essential for any systematic approach to the modeling of biological systems.
Protein function prediction methods are techniques that bioinformaticians use to assign biochemical roles to proteins.
By using various machine-learning approaches, we work on the development of predictive models for Enzyme Commission number (E.C. number). ECpred classifies an enzyme in one of 3349 EC numbers. |
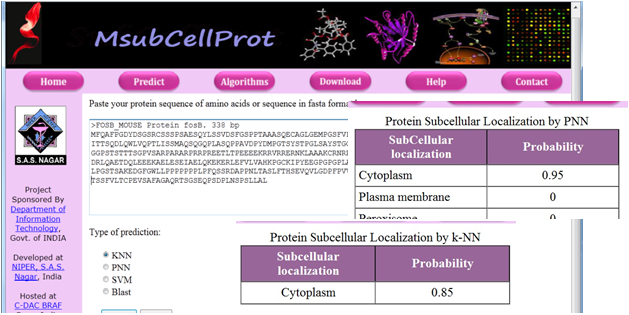 | Among the various
approaches to decipher the function of a protein, one is to
determine its localization. Two machine learning algorithms, k Nearest
Neighbor (k-NN) and Probabilistic Neural Network
(PNN) were used to classify an unknown protein into one
of the 16 subcellular localizations. The final prediction is
made on the basis of a consensus of the predictions made
by two algorithms and a probability is assigned to it. The
results indicate that the primary sequence derived features
like amino acid composition, sequence order and
physicochemical properties can be used to assign
subcellular localization with a fair degree of accuracy.
|
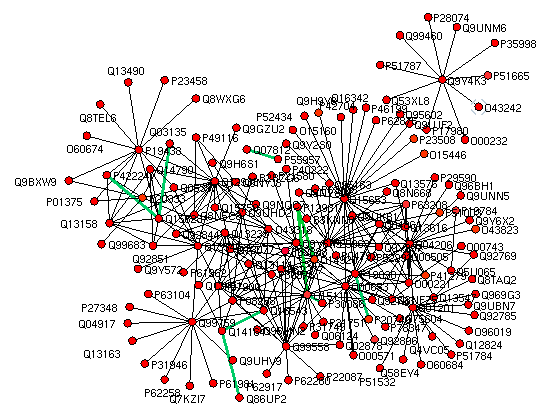 |
Protein-protein interactions (PPI) are molecular basis of most of the cellular processes in a biological system.
It is necessary to study these interactions at global level, as a network to actually understand biological processes,
where proteins interact with each other to carry out verity of physiological responses. Here, we have presented an in silico approach using machine learning
method for PPI prediction using primary sequence information and associated physicochemical properties of constituting amino acids. SVM algorithm was used
to recognize patterns in these sequences to make a statistical decision as to whether or not a query protein pair will interact.
|
Adverse Drug Reactions |
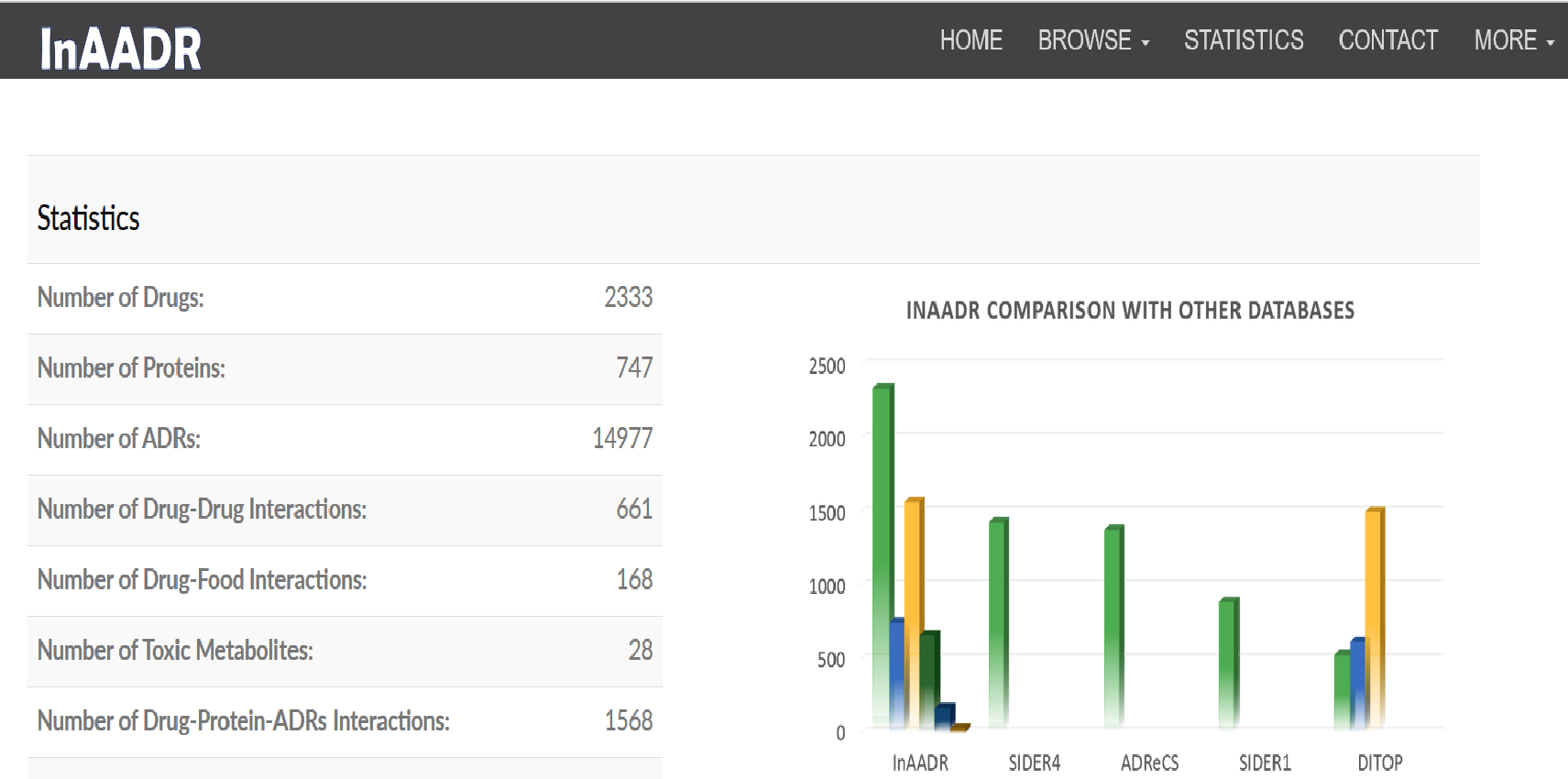 |
InAADR (Interactions Associated with Adverse Drug Reactions) database provides drug-protein-ADRs, drug-drug and drug-food interaction information and their associated side effects.
The database may be accessed from http://14.139.57.41/InAADR/.
|